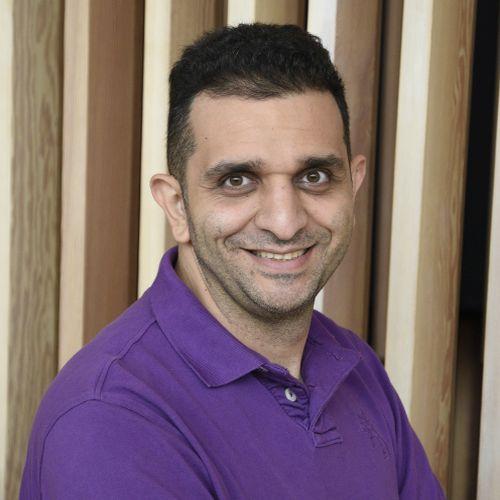
Nasir, Vahid
- Smart Data-Driven Manufacturing
- Applied machine learning & AI
- 3D Printing
- Industry 4.0 / Industry 5.0
- Human-centric design and manufacturing
- Sustainable and resilient production systems
- Renewable materials for innovative product development
- Process condition and health monitoring
-
WSE 461/561Introduction to Wood Products ManufacturingFirst of a three-term series exploring technologies and management practices associated with manufacturing products from wood and other renewable materials. Covers major processing steps for the conversion of raw materials into products. Emphasizes major processing steps, equipment, and the impact of material properties on processing strategies.
-
WSE 463/563Advanced Manufacturing 2Third of a three-term series exploring technologies and management practices associated with manufacturing products from wood and other renewable materials. Subjects covered include process control, optimization, automation, and contemporary topics such as Big Data and the Internet of Things and the potential impacts of the trends on manufacturing enterprises.
Gyawali, B., Haghnazar, R., Acula, P., Laba, K., Nasir, V., A review on 3D printing with clay and sawdust/natural fibers: printability, rheology, properties, and applications, Results in Engineering, 103024.
Haghnazar, R., Ashjazadeh, Y., Hauptman, J., & Nasir, V. (2024). A Computational Design Integrated Digital Fabrication Framework for Mass Customization in Industry 5.0 Manufacturing with Non-Standard Natural Materials. Results in Engineering, 102400.
Nasir, V., & Sassani, F. (2021). A review on deep learning in machining and tool monitoring: Methods, opportunities, and challenges. The International Journal of Advanced Manufacturing Technology, 115(9), 2683-2709.
Nasir, V., Dibaji, S., Alaswad, K., & Cool, J. (2021). Tool wear monitoring by ensemble learning and sensor fusion using power, sound, vibration, and AE signals. Manufacturing Letters, 30, 32-38.
Schimleck, L., Ayanleye, S., Avramidis, S., & Nasir, V. (2023). A chemistry-based explainable machine learning model based on NIR spectra for predicting wood properties and understanding wavelength selection. Wood Material Science & Engineering, 18(6), 2116-2127.
Nasir, V., Ali, S. D., Mohammadpanah, A., Raut, S., Nabavi, M., Dahlen, J., & Schimleck, L. (2023). Fiber quality prediction using NIR spectral data: tree-based ensemble learning vs deep neural networks. Wood and Fiber Science, 55(1), 100-115.